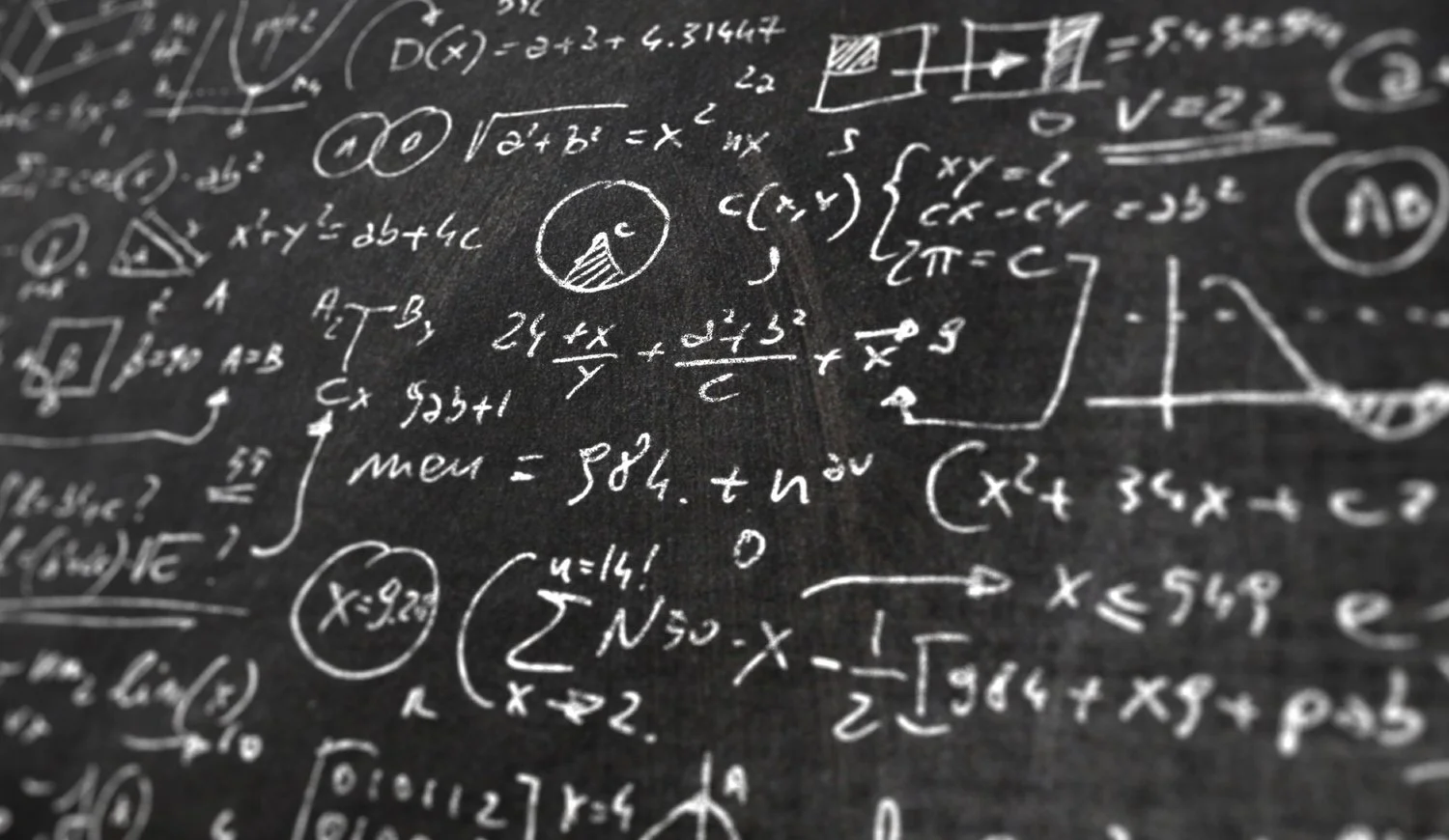
Comparing single outcome and variable
Researchers
Why is it important?
One of the most basic aspects evaluating improvements in healthcare or surgical techniques is by comparing the outcomes of two (or more) different procedures.
How is it done?
Like summary measures, statistical tests used to compare outcomes are based on calculations that make assumptions on the data distributions. Therefore it is important that the correct test is applied to the corresponding data distributions. In general, when comparing two different and independent outcomes, a t-test is used to compare normally distributed continuous data, the Mann-Whitney or a Wilcoxson rank sum test is used to compare continuous non-normally distributed data and the Chi-square test (or Fisher’s exact test if the numbers are very small) is used to compare categorical data.
The statistical test generates a P value for example 0.05, which correctly interpreted means that if the test was done (over and over) many times, the likelihood of observing the difference (or more extreme value) due to chance is 5%.
What is the relevance?
It is important to use the correct test to each distribution, for example if a Chi-square test is used when the observed (more correctly predicted) values are very low (e.g. 1/25) then the chances of achieving a statistically significance result is (incorrectly) easier.
Many clinicians do not understand how to interpret a P value. Firstly, it implies that the “test” will be performed over and over again many time (long run frequency), and this is clearly not the case in clinical practice. Secondly, many take it as an absolute value in that a P-value of 0.04 is significant but a P value of 0.06 is not. To appreciate that attitude is saying that a 4% and 6% chance of rain tomorrow is extremely and completely different, clearly for all intents and purposed there is no difference between 4-6%. In fact, my own opinion is that there really is not much difference between 5 or 10% (i.e. P=0.10). Another lesser known fact is that the P value is driven by the size of the data, therefore differences between 9/10 versus 7/10 may not be significant (P=0.582), but the P value for the difference between 9,000/10,000 versus 7,000/10,000 is (P<0.001), this becomes more important to appreciate when numbers are large – all differences (whether clinically important or not) become significant.
If you find this type of teaching useful and would like to learn more, I run an online statistics course for clinicians and researchers: